A Conceptual Model-driven Method for Big Data applications
The term Big Data is increasingly present in the development of software applications and services on different application areas such as health or digital economy. The term is usually associated to technological concerns, related with solutions that manage and physically store big volumes of data. This interpretation has caused a proliferation of isolated Big Data technological solutions, generating a huge data chaos. However, a high quality technological infrastructure is not enough if it lacks the suitable mechanisms to organize and extract value from the stored data.
This project focuses on analysing, formalizing and solving conceptual and methodological challenges that arise while developing applications and services based on Big Data in industrial environments. Starting from an ontology that describes this domain without ambiguities and applying conceptual model-driven software development (MDSD) principles, we propose a conceptual model-driven method for developing Big Data applications (DataME). The goal is defining precise and rigorous conceptual models that drive the development of Big Data applications and services in order to provide business value. This way, we introduce the enterprise perspective without focusing on technological parameters of performance and scalability. In order to define this method, we will face the next four relevant scientific challenges:
(C1) In order to ensure value, we must establish a precise conceptualization about which information is relevant for organizations. This step is usually avoided, leading to technological solutions that do not fit their needs. From the methodological point of view, aligning organizational goals with technological solutions is essential.
(C2) Retrieving relevant knowledge from big volumes of data is only feasible after solving the heterogeneity among the different data sources. However, this integration must ensure data quality to avoid the generation of incorrect knowledge. In order to address this challenge, we will propose a conceptual alignment strategy that ensures the quality of the integrated information and allows the usage of relevant information as a whole.
(C3) Detecting and selecting relevant knowledge from Big Data volumes is only possible with interaction mechanisms that allow the final user searching and accessing information easily and precisely. Identifying this kind of interactions also requires a conceptual model perspective in which domain concepts guide the data operations that will be able to give value to the expert user.
(C4) Ensuring results quality and precision requires automatic testing methods working on highly distributed environments. Without this quality, Big Data can become into “Wrong Data”, disrupting the knowledge obtained. In order to address this challenge, we see a huge potential in using the testing automation tool TESTAR (testar.org), a result of the European project FITTEST (Future Internet Testing).
The DataME method will provide a holistic solution to these four challenges. As a proof of industrial application, we will apply this method in the development of a Big Data application for the management of genomic data in several organizations of the field.
Main Researcher:
Óscar Pastor López
Period:
2018-2020
References:
TIN2016-80811-P
Funding Organization:
Ministerio de Economía y Competividad
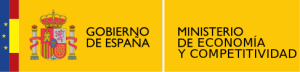
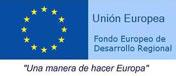